Damaged roofs, uprooted trees, widespread flooding were among the scenes on the path of Hurricane Maria, a Category 5 hurricane that slammed Dominica and Puerto Rico and has caused at least 78 deaths (as of October 1) including 30 in Dominica and 34 in Puerto Rico, and many more left without homes, electricity, food, and drinking water. Below are some of the images we collected from Twitter show devastation due to Hurricane Maria.
At the onset of a disaster like Hurricane Maria, humanitarian organizations such as United Nations Office for the Coordination of Humanitarian Affairs (UN OCHA), government agencies, public health authorities, and military are tasked with responsibilities to save lives and reach people who are in need of help. Situation-sensitive requirements arise during such events and formal disaster response agencies look for actionable and tactical information in real-time to effectively estimate early damage assessment and to launch relief efforts accordingly. In recent years, we have witnessed the widespread usage of social media and microblogging platforms such as Twitter and Facebook, during major events such as Arab Spring and Boston bombing. These online communication channels provide rapid access to situational and actionable information from eyewitnesses and affected people during natural hazards. Research studies have revealed that a variety of information on social media during disasters can be useful to gain situational awareness and to understand urgent needs of the affected population such as requests for food, water, shelter, and medical assistance.

For rapid crisis response, real-time insights are important for emergency responders. To identify actionable and tactical information pieces from a growing stack of social media data and to inform decision-making processes as early as possible, messages need to be processed as they arrive. Given the high volume of social media messages, we need to triage them by categorizing them into different actionable bins such as food, supplies, financial, logistics, etc., which is exactly the purpose of the Artificial Intelligence for Digital Response (AIDR), a technology developed by the Qatar Computing Research Institute (part of Hamad Bin Khalifa University). AIDR uses supervised machine learning techniques to classify each message/tweet as it is posted on social media. AIDR is capable of classifying both textual messages and images in real-time.
We activated AIDR on September 20, 2017 to collect tweets related to Hurricane Maria. Since then more than 2 million tweets have been collected (as of October 6, 2017). Figure 1 shows the distribution of the daily tweet counts. To understand what these tweets are about, we applied our tweet text classifier which was originally trained (F1=0.64) on more than 30k human-labeled tweets from a number of past disasters. Figure 2 shows the distribution of tweets into different classes. As one the most prevalent categories during emergencies in general, the “Not related or irrelevant” message category contains around 28% of the whole data in the Hurricane Maria case. The “other relevant information” category emerged as the second largest category, which is also not surprising. Research studies have found that this category contains from about 10% to 30% of the messages. For more information about why AIDR uses this category and why this category could be useful to learn about several small-scale unanticipated events, you can read these papers: (Imran & Castillo, SWDM 2015) and (Imran et al., ICDM 2015).
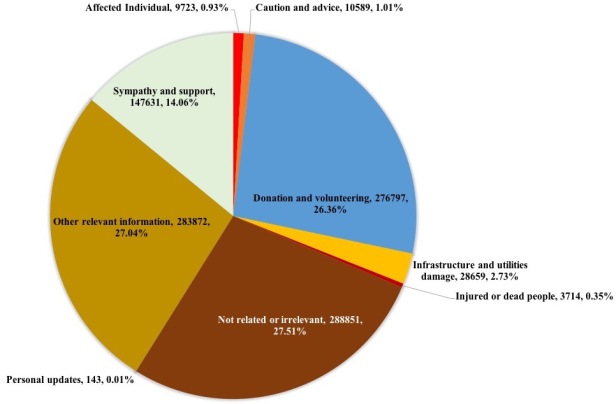
In the remaining categories, similar to our Hurricane Harvey results, the “donation and volunteering” and “sympathy and support” contain substantial amounts of messages, specifically 26% and 14%, respectively. Even after the automatic classification of tweets and filtering-out irrelevant ones, the remaining messages are still in thousands. Manual analysis of these thousands of messages (e.g., ~27k donation-related messages) is a hectic and time-consuming task for crisis responders, especially during an on-going disaster event. To overcome this information overload issue by helping crisis responders sift through enormous data as quick as possible, we at QCRI are working on building innovative technologies. Our recent work “Interactive Monitoring of Critical Situational Information on Social Media” aims to solve these issues through an interactive dashboard. This technology is currently under testing and hence not available for public use. However, we deployed the dashboard to analyze the Hurricane Maria’s data. Figure 3 shows the interactive dashboard and its different visual components including timelines (top), machine classifiers (left), top critical entities (people, organizations, locations), and tweets view (bottom).
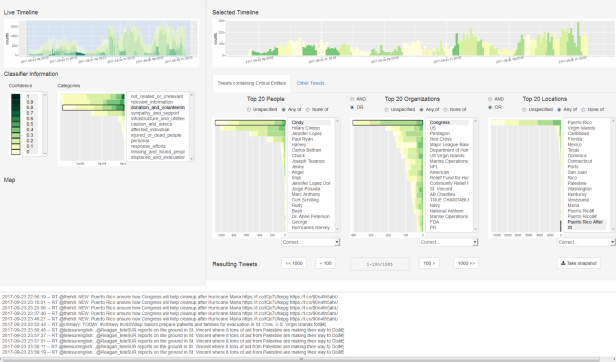
We use the Stanford Named Entity recognition tool to extract the critical entities that are being mentioned in the tweets using tweet content. One can apply different combinations of filters to analyze thousands of tweets in real-time. For example, in Figure 4, we selected “Carlos Beltran” from the top 20 people mentioned in the “Donation and volunteering” category to check why this person was being mentioned. And all the tweets with a mention of the selected entity appear in the tweets panel (bottom). In this particular case, we see several tweets around the news that “…Carlos Beltran and his wife have started a Hurricane Maria relief fund for Puerto Rico with a $1 million donation…”.

By further analyzing the data, we found other important tweets, which are listed below with their corresponding categories. The first two tweets under the “Donation and volunteering” category clearly specify urgent needs of the affected people in Puerto Rico. Automatic extraction and consolidation of these urgent needs in real-time would be hugely useful for crisis responders. Tweets #3, 4, and 5 emphasize the need for help. In the “Infrastructure and utility damage” category, the tweet #3 does not only report about a damaged dam but also emphasizes on the fact that 70k people are at risk. Detecting such information about damaged infrastructure which is likely to bring severe consequences either in terms of human lives or more economic damage help concerned authorities to determine the level of urgency to act. Similarly, not to mention, how important are the two tweets reporting missing people in the “Missing and found people” category. Despite, almost half of the data we collected on Hurricane Maria is irrelevant, there exist diamonds in the rough which can be useful for a number of crisis response and management purposes.
Donations and volunteering requests or offers:
- RT @DorothyKidd1: Food, water, power, gasoline desperately needed in Puerto Rico https://t.co/z3tVTc7pAU
- Puerto Rico needs our help. Bring diapers, baby food, batteries, first aid supplies, feminine hygiene products to:… https://t.co/KWi6uCWMxz
- Our neighbors need our help! Click on the link on our page and donate what you can. Thank you! https://t.co/zyITNsMUHy
- Frustration mounts in Puerto Rico as people in need wait for relief supplies https://t.co/Rnk4fRuZML via @cbsnews
- RT @PatriceMusik: We need your help. Please donate to our LifeLink of Puerto Rico Staff Aid. #HurricaneMaria #ourstaff #donate https://t…
- Many PRworkers left w/o house, power, water, food, comms, transp, gas. That’s why help is needed. @realDonaldTrump… https://t.co/tW06yk7fKI
Infrastructure and Utility Damage reports:
- Bavaro, Dominican Republic A gift shop on Cofrecito beach is damaged after being hit by #HurricaneMaria Photograph:… https://t.co/51CmpqAtG2
- @Reuters Destroyed homes are seen from a Marine Corps Osprey surveying damage from #HurricaneMaria in St. Croix,… https://t.co/T7Xtc8vslP
- RT @youthmappers: There are 70k people downstream of this dam damaged by H.Maria https://t.co/cyki5UMZyq
- Hurricane Maria leaves Arecibo radio telescope damaged and dark https://t.co/b1G7EW9BEI via @theregister
- Hurricane Maria Damages Dominica’s Main Hospital, Leaves ‘War Zone’ Conditions https://t.co/JuCd4XIkW8
- RT @NWSPittsburgh: Maria did a lot of damage to our Doppler Radar in San Juan, PR. Here is what a radar should look like.…
- San Juan airport remains crippled by Hurricane Maria damage https://t.co/167dRw8OkX
- Maria’s Devastation Damaged Hospitals Running Out of Supplies in U.S. Virgin Islands @weatherchannel https://t.co/BWfZmCRU1y
Missing or found people reports:
- RT @Jasamsdestiny: Amy is missing – plz help find her. #Miami #southmiamiheights #palmettoestates #miamidadecounty #MiamiBeach…
- #hurricanemaria QUESTELL FAMILY MISSING 5 DAYS IN PONCE. PLEASE HELP FIND THEM
AIDR’s image processing pipeline was also activated to identify images that show infrastructure damage due to Hurricane Maria. Around 80k tweets contained images. However, ~75% of these images were duplicate. The remaining 25% (~20k) images were automatically classified by the AIDR’s damage assessment classifier into three classes namely SEVERE damage, MILD damage, and NO damage. Figure 5 shows the distribution of images into these three classes. Almost 78% of the images we collected on Twitter do not show any damage, mainly because these images depict individuals or infographics rather than built structures. However, 10.9% of them show MILD damage and 11.2% of the images show SEVERE damage.

Below we show example images from the three damage classes which were automatically classified by our deep learning damage assessment classifier.
Results of the damage assessment classifier (Click to enlarge)
By looking at the actual image content, we can extract more information about the devastation caused by the disaster than relying solely on the textual content provided by the users. Even though it is in the testing phase, our image processing pipeline does a decent job in identifying images that show MILD or SEVERE damage. Instead of trying to look at all the images, humanitarian organizations and emergency responders can simply take a look at the retained set of MILD or SEVERE damage images to get a quick sense of the level of destruction incurred by the disaster.
Related papers:
- Muhammad Imran, Carlos Castillo, Ji Lucas, Patrick Meier, and Sarah Vieweg. AIDR: Artificial Intelligence for Disaster Response. In Proceedings of the 23rd International Conference on World Wide Web (WWW) Companion, 2014. Seoul, Korea.
- Dat Tien Nguyen, Ferda Ofli, Muhammad Imran, Prasenjit Mitra. Damage Assessment from Social Media Imagery Data During Disasters. In Proceedings of the IEEE/ACM International Conference on Advances in Social Networks Analysis and Mining (ASONAM), 2017, Sydney, Australia.
- Firoj Alam, Muhammad Imran, Ferda Ofli. Image4Act: Online Social Media Image Processing for Disaster Response. In Proceedings of the IEEE/ACM International Conference on Advances in Social Networks Analysis and Mining (ASONAM), 2017, Sydney, Australia.
- Michael Aupetit, Muhammad Imran. Interactive Monitoring of Critical Situational Information on Social Media. In Proceedings of the 14th International Conference on Information Systems for Crisis Response And Management (ISCRAM), 2017 Albi, France.